When Orville Wright covered 36 metres aloft a field in Kitty Hawk 120 years ago, it was a milestone. A moment. One that we can all recognise today as the point where heavier-than-air flight becomes a reality. But what could one achieve with a single-seat, bicycle-wheeled device? Not much in truth. No cargo capacity, no range, no passengers, and fragile, damaged beyond repair after its first day.
And yet, it marked a milestone in technological development.
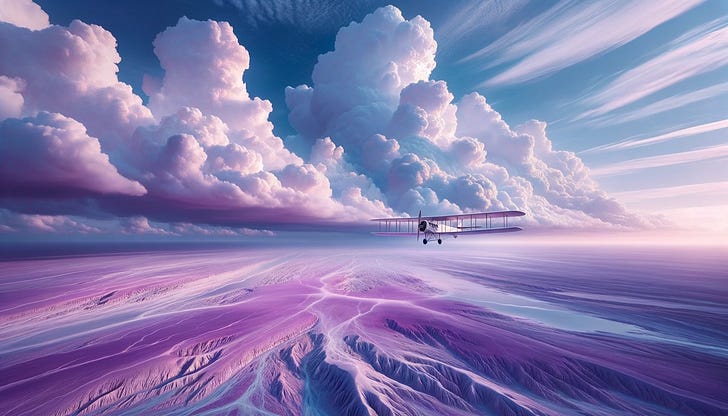
There is a telling line in a recent essay written by Peter Norvig and Blaise Agüera y Arcas. They write:
Decades from now, [today’s advanced LLMs] will be recognized as the first true examples of AGI, just as the 1945 ENIAC is now recognized as the first true general-purpose electronic computer.
And as the Wright Flyer was for aviation.
Norvig and Agüera y Arcas sum up something that I have felt, shared in essays and spoken about to many of you over the past months. They explain how these emerging models are showing a general capability across tasks, domains, media types, and even controlling robotic actuators. Not “perfectly” but there appears to be an early direction..
The vast variety of tasks I have put GPT-4, ChatGPT, Claude, and Perplexity through is almost inestimable. These systems have helped me across a wide variety of tasks, including:
Planning the acoustic panelling in my office.
Analysing my Oura, CGM, and Apple Health Data to understand patterns and trends.
Assisting with research tasks for my new book.
Reviewing drafts of speeches and suggesting improvements.
Helping me understand the difference between balanced and unbalanced headphone connections.
Briefing me on different measures of market concentration.
Helping to understand some of the wider impacts of semaglutide on other conditions.
Helping me figure out how to install unapproved extensions on my Mac.
Finding and reviewing news stories about data centre energy usage.
Assessing the currency performance of JPY, EUR, USD, and GBP.
Helping me understand what monoclonal antibodies could be used to treat asthma and what the patient profile of these might be.
Challenging the analogies I used to describe energy usage through the lens of the principles of entropy.
Contextualising the musical heritage of DJ Shadow.
Grading my work and finding weaknesses in my arguments.
Helping draft prompts for other LLMs or image generators, like Midjourney.
Helping me organise the books on my bookshelves.
And many, many more.
Good enough, given the alternative
Not all of these tasks were completed perfectly. With the bookshelf task, GPT-4 came up with six excellent categories (and about a dozen sub-categories) for a selection of around 100 books. But it only went on to organise about half of them. The model was absent minded and forgot half of my books. As I prompted it to remember them it wandered down a hallucinatory maze. Yet, even that half job saved me hours.
For many of the tasks, I’d judge that one of these primordial LLMs performs about as well as I (or the people I work with might). I say about as well because at times they just fail. But having worked with hundreds of people over the past 25 years, for a really wide variety of tasks, GPT-4 or Claude can do as well as humans, even those who have been through a recruitment process.
In my career, I’ve often had the sort of white-collar job that can only exist in a large firm: writing competitive analysis or market trends; prepping the C-level for board meetings; producing product documentation; and summarising analyst reports. In startups - where I spent nearly two decades - as a founder, product manager, marketer, dogbody, you name it.
In both of those roles, big tony firms with business-class travel policies and scrappy startups in crumbling shared offices, I did plenty of work that today’s LLMs could really help with: analysing qualitative user interviews to help understand user personas; needs and segments; helping assess product specifications; evaluating technical choices; drafting minutes of meetings; helping with investors’ letters… the list goes on.
And here’s the thing: true for me and those who worked with me — we, as humans, didn’t always get it right. Product documents might go through iteration after iteration. A first, even second draft, of a market analysis might still need multiple iterations to be good enough. Even highly trained humans. The outputs from today’s LLMs, in conjunction with a human operator, in some tasks, compete with the quality of human teams.
When testing a computer system’s performance on a knowledge task, what is the best benchmark for comparison - the top human expert in that field, someone in the top 25% of human proficiency, or the average human ability? My sense so far, which researchers will analyse more formally, is that these AI systems perform at or above the level of a smart human across many knowledge tasks. We might complain that ChatGPT only answers at an undergraduate level, but most humans can’t answer questions about biology, medieval England or VPN set-ups at an undergraduate level. So they seem to be doing something above and beyond a Google search or pivot table.
If that weren’t the case, I wouldn’t have swapped many of my Google searches for Perplexity queries. A simple fuzzy question to Perplexity — where I can barely express myself — often yields better answers than iterating query after query on Google.1
In our regular “AI in practice” sessions for annual premium members, we’re cataloguing dozens of powerful uses of these technologies — the next session is on 26 Oct.
In the rest of this essay, I explore:
What should we make of the scientific and theoretical objections to current LLMs?
What is the the extent to which LLMs are becoming integral to our modern economies?
What are the implication of AI as a GPT machine tool?
What does it mean to have a society of AI?