Hi, it’s Azeem. Here’s a shocking reality check: every major energy forecaster has been wrong about solar power uptake. So wrong, in fact, that when I recently offered a $1,000 bet on solar’s explosive growth, not a single expert would take me up on it. This isn’t just a forecasting error – it’s a systematic blind spot.
To understand this phenomenon, I turned to someone who’s watched it unfold from the inside:
. Nat spent almost a decade at the intersection of energy, technology and climate. He joined New Energy Finance, the leading market researcher looking at the energy transition, when it was just a startup. He worked there through its acquisition by Bloomberg, when it became BloombergNEF. Most recently Nat co-founded Halcyon, an AI-assisted research and information platform focused on energy transition and decarbonisation. All in all, he knows what’s going on – and why forecasters keep missing to see exponential trends.Here’s Nat’s view, exclusively written for Exponential View members.
The forecasters’ gap
By Nat Bullard, co-founder of Halcyon
Every year this decade, the International Energy Agency (IEA) has had to revise its solar deployment forecasts upwards. And not just by small amounts. Actual installations have been as much as three times higher than the agency predicted five years ago.Â
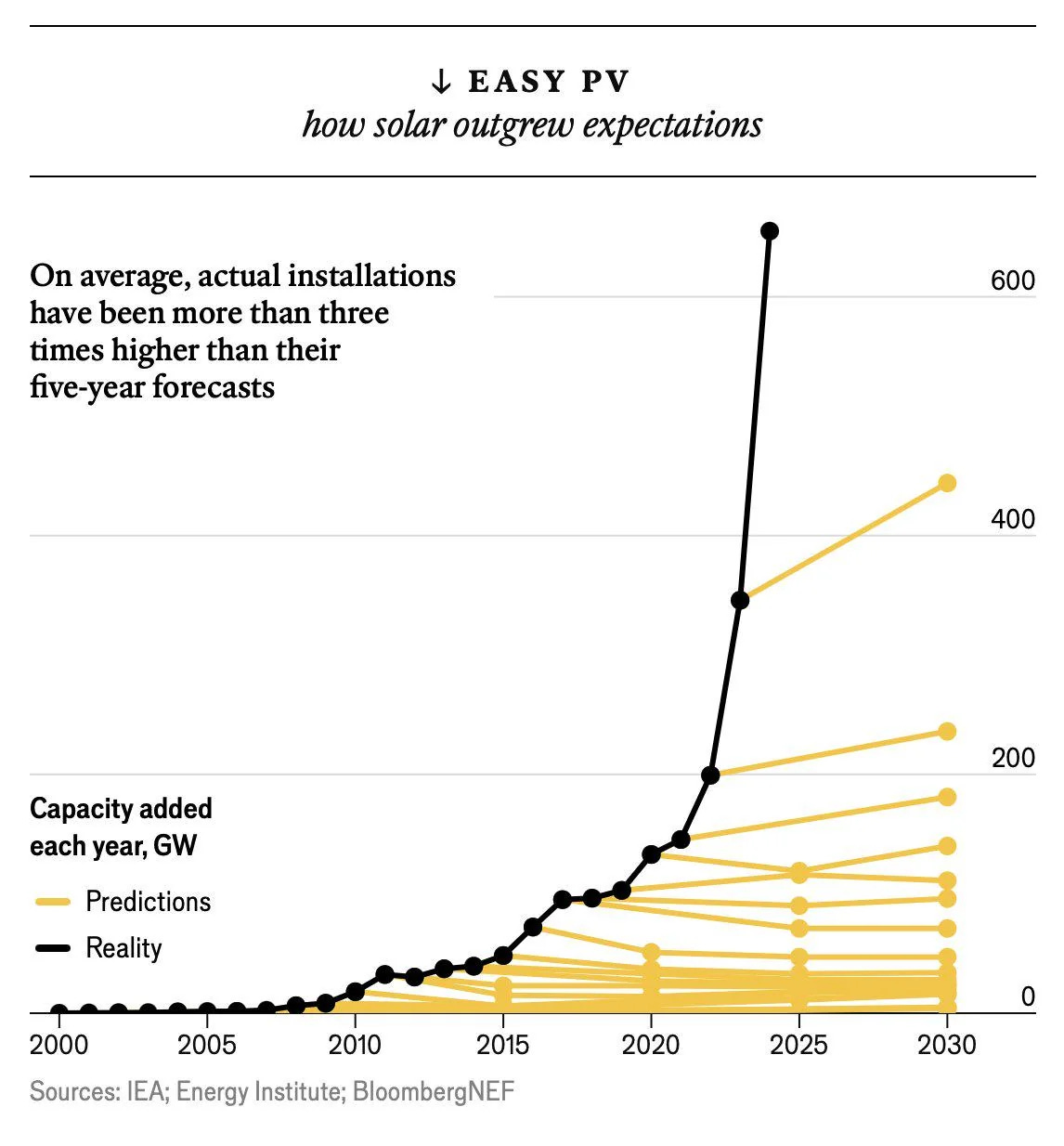
But it’s not just the IEA. Almost every organisation that has tried to look at the longer-term future of solar power globally has undershot, often significantly. So, what’s going on?
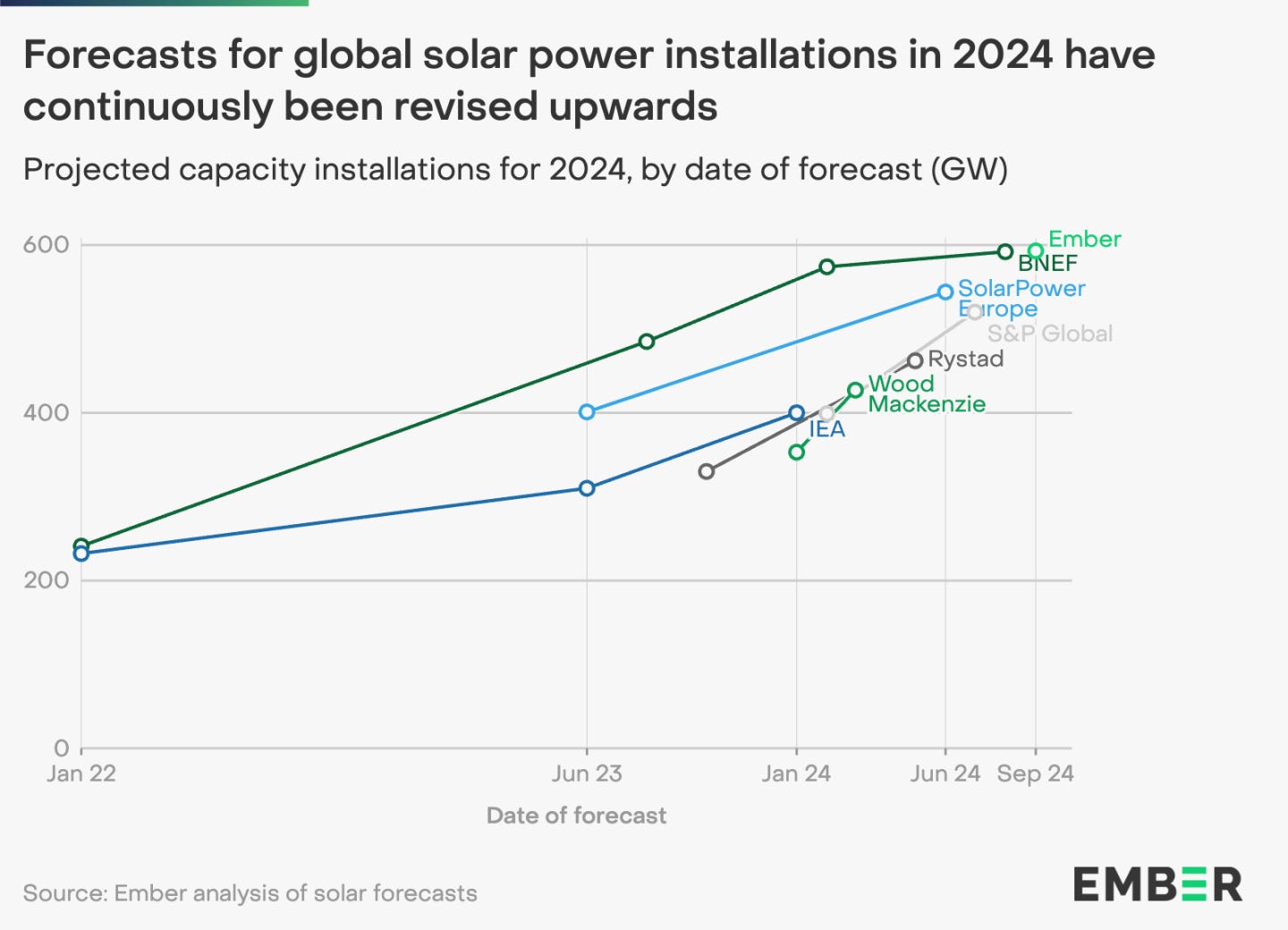
A tale of two futures
A few weeks ago, Azeem made a bet: in 2027, global solar photovoltaic additions will be no less than 975 gigawatts. No forecaster has taken up the challenge of disputing this forecast yet. It seems that even those who routinely make conservative predictions are aware of their limitations. If all the analysts know that these forecasts are habitually low, why do they remain unchanged?
It’s because they don’t make predictions in the way that we think they do. People often conflate two distinct types of forecasting, each with its own logic, inputs and purpose.
The short-term forecast is truly a fore-cast: a short throw into the future. It is similar in this respect to a weather forecast. It’s based on concrete, observable data and current trends, the more certain the closer it is to the present, the less certain the further out. The components of this view of the future are all quite empirical: customer behaviour patterns, recent market movements or immediate economic indicators.
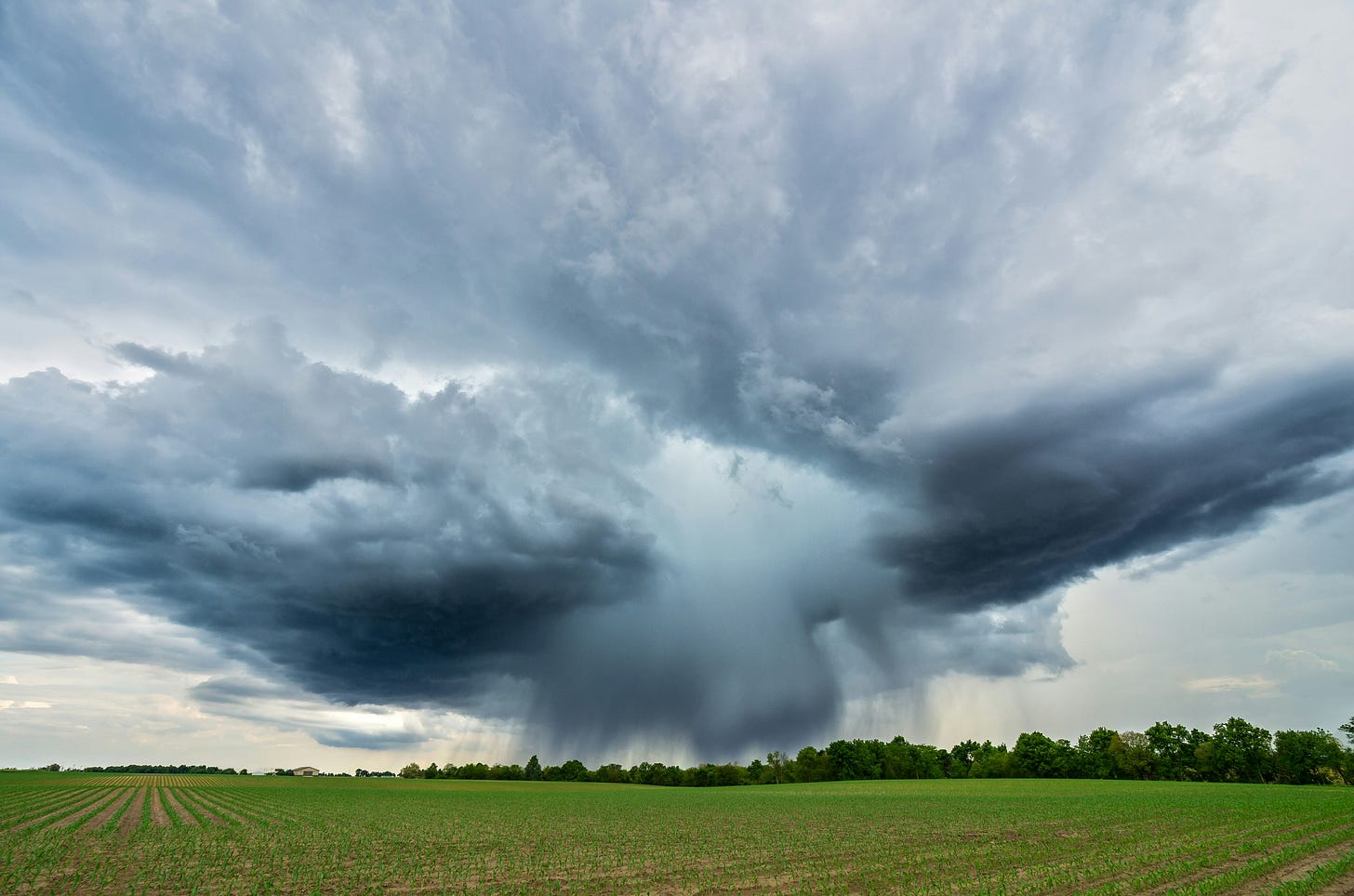
A long-term forecast is fundamentally different from these short-term predictions.1 While some might call it a forecast, it’s more accurately described as a projection into the future. Unlike short-term forecasting’s empirical focus, a long-term projection combines current data with a broader theory of change that seeks to understand how and why systems evolve.
Rather than simply extending current trends, it attempts to identify the underlying forces and mechanisms that drive transformation. Think of it like predicting climate change rather than weather – you’re examining deeper systemic factors and potential paradigm shifts.
The crucial insight is that most energy industry forecasts, even those projecting years into the future, are essentially short-term forecasts stretched beyond their useful range. They rely primarily on current observable data and trends, and are therefore inherently limited in their ability to capture the complex, dynamic nature of long-term change.Â
Who needs short-term forecasts?
Short-term forecasting is the type of work that client-paid research providers like BloombergNEF, Rystad & Wood Mackenzie produce regularly. They are paid to provide practical forecasts for business decisions. Clients need immediate, practical insights, such as how much solar capacity would be built in the Mid-Atlantic region of the US over the next few years. These companies rarely seek long-term predictions – focusing on the next page, not the next chapter. They want to know where to allocate resources in the near term.
However, given that solar forecasts are so often wrong, wouldn’t this cause chaos for businesses working with the wrong data?
It can have an impact, but the outcome depends on other factors, too, such as the tightness of the supply-demand balance, for example. It is almost irrelevant if a short-term view of global solar installations – used to inform a solar module pricing strategy – misses the reality by 5-10% on the downside, if the market is 100% oversupplied with solar modules.
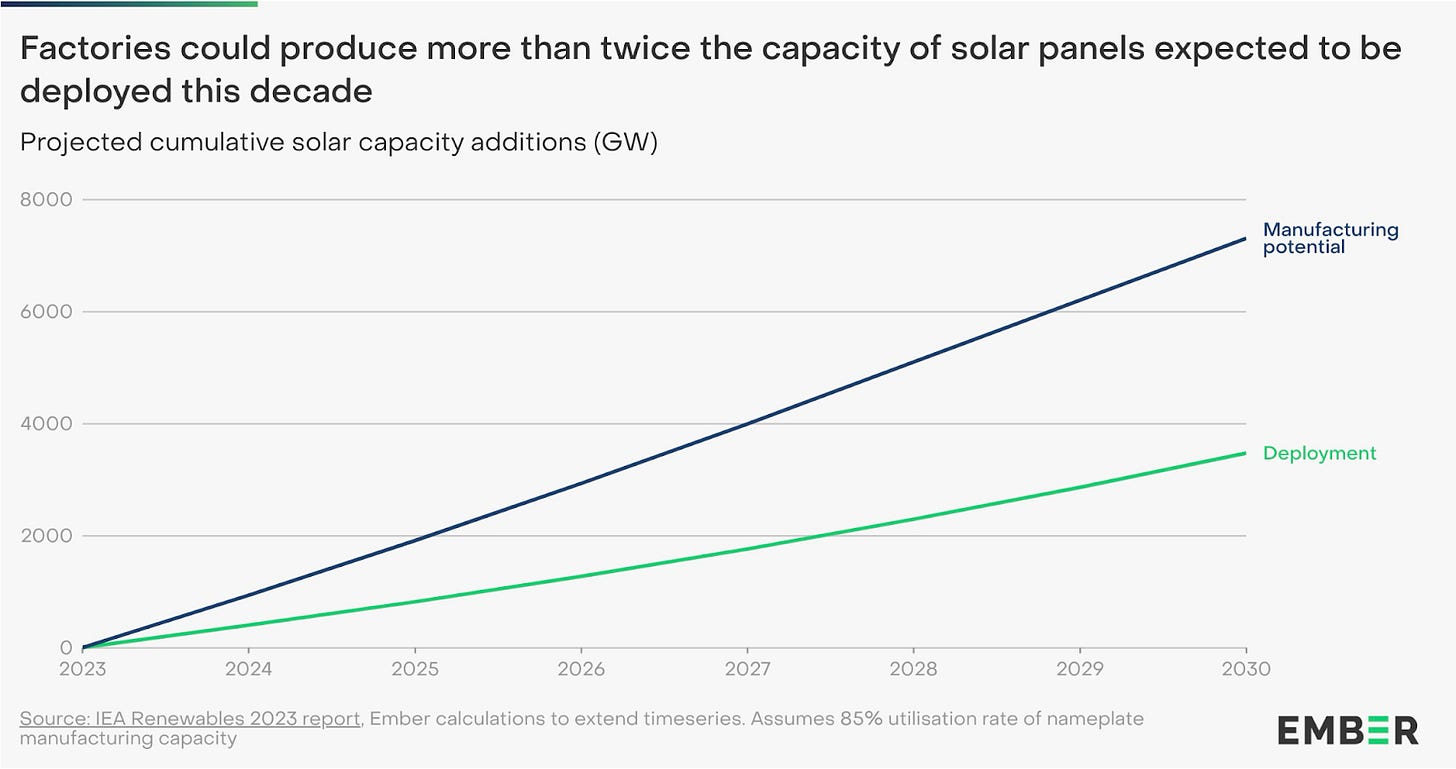
In some cases, when short-term forecasters observe a change in the data, they change their forecasts. A great example of this is Pakistan’s solar boom. The country’s demand for grid electricity fell by 9% year-on-year due to a surge in solar adoption – a shift absent from any major forecast. Forecasts were then revised because the data was there to prove it was happening. It was not possible to predict this surge before, because it was contingent upon many factors that had to come together for it to happen: the unique structure of Pakistan’s electricity market, favourable pricing for photovoltaic modules, and a supply landscape that let companies serve Pakistan without undermining their other markets etc.Â
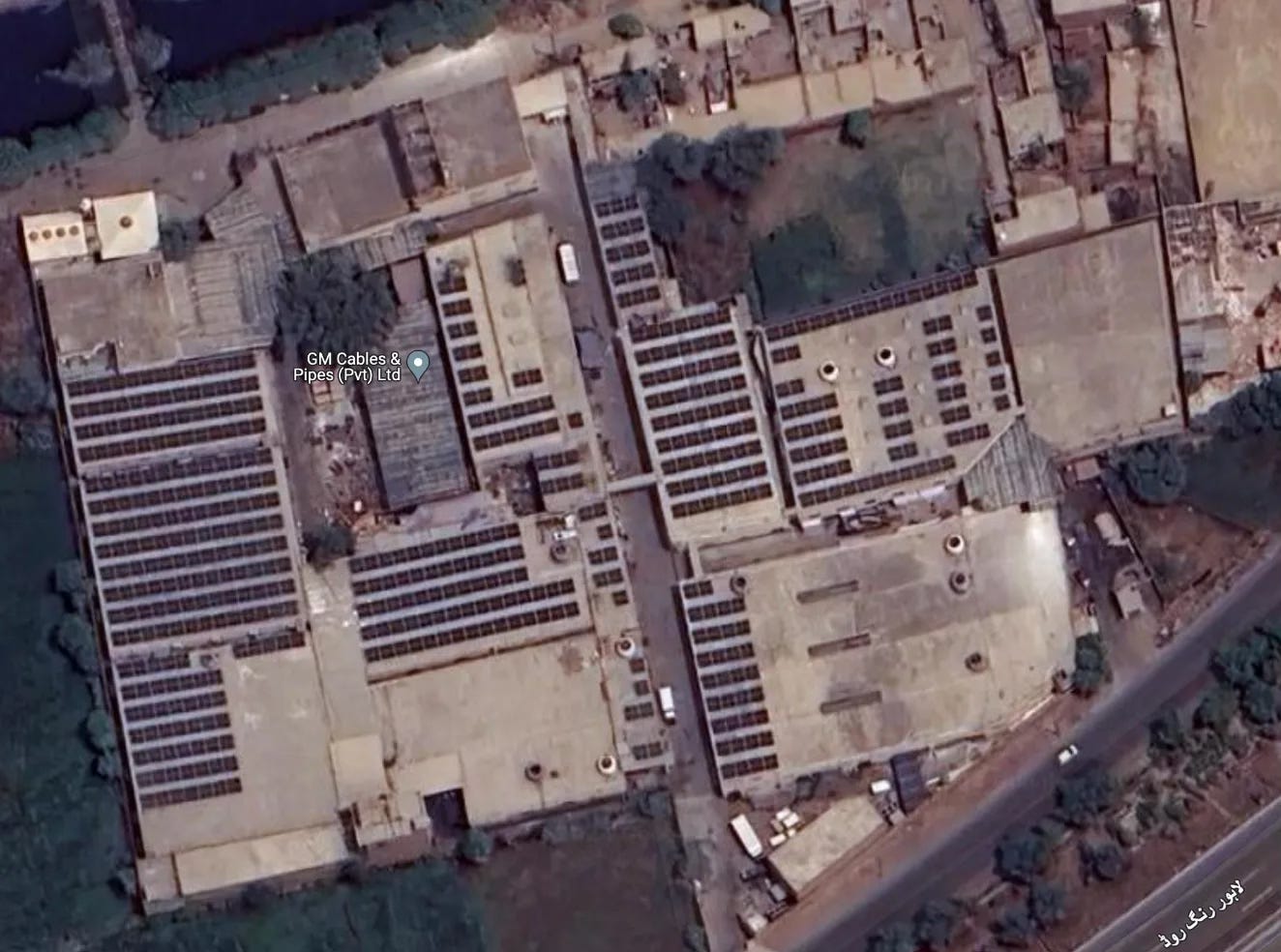
Another question to consider is: which individuals or institutions would pay for exceptional accuracy in the short term? Pakistan’s national electricity provider doesn’t need an extremely granular forecast to recognise a material change: it’s evident in its own operational data. What they need is help in managing the impact of that unexpected shift. This kind of projection rises (or falls) to accommodate what is being observed. It’s not a theory of change; it’s an observation of trends for which there isn’t really a theory.
How long-term forecasting shapes markets
What has proven to be more accurate for predicting the solar industry is long-term forecasts, which incorporate a thorough but supple theory of change. As a reader of Exponential View, you know this well. Simple, extrapolative heuristics based on fundamental drivers have proven more accurate for predicting solar deployment than complex forecasts. It’s similar in reasoning to the success of Moore’s Law – where a simple observation about transistor density doubling every two years proved remarkably predictive, because it was anchored in fundamental manufacturing improvements. Similarly, fitting a logistic curve to solar adoption typically works better than elaborate models because it captures the underlying drivers: manufacturing scale, technological learning and cost reduction. These fundamentals create a cascade of predictable market responses at the macro level.Â
To return to Pakistan as an example…
Keep reading with a 7-day free trial
Subscribe to Exponential View to keep reading this post and get 7 days of free access to the full post archives.