AI’s productivity paradox: how it might unfold more slowly than we think
From steam engines to GPTs: the long road to productivity
I want to play a game of counterfactuals. If artificial intelligence disappoints as a technology, why might that be?
Historically, technologies like the steam engine and personal computers boosted productivity, raised GDP and reshaped societies—transforming where and how people live, work and even what they value. I believe AI is such a technology and it has the potential to deliver those benefits—and faster than previous technologies.
But, of course, I always like to challenge my own thinking.
So, today I want to explore a scenario where AI disappoints by failing to deliver significant productivity improvements within a short timeframe—say, five years. Through this lens, I’ll examine the challenges that could slow down AI’s impact and think about the implications of a less revolutionary trajectory.
AI as a GPT
Before we discuss potential disappointing scenarios, I want to first lay out the reasons that AI could be a general-purpose technology.
First of all, AI’s potential as a GPT lies in its versatility. GPTs are broadly applicable across sectors, improve rapidly, spur complementary innovations and fundamentally reduce the cost of critical economic activities. Unlike specialized technologies, AI can tackle a vast array of tasks—from summarising emails to designing board games, from writing code to finding new molecules.
Its rapid progress is impressive: large language models have evolved from producing short text fragments to tackling math problems at the level of the top 0.1% of high school students.
AI also fosters complementary innovations. Downstream, we are seeing huge innovations in hardware; performance per dollar of Nvidia GPUs has been improving by around 30% per year. Consider ASICS like Cerebras and Groq or the resurgence of interest in reversible computing.
Focus should go beyond the chips... Just look at what is happening in bandwidth. We have moved networks from 10 megabit ethernet in the 1990s to 1.8 terabit NVLink today – 180 thousand times the speed. And don’t forget innovations in cooling — such as immersion cooling or the use of microfluidics to cool chips.
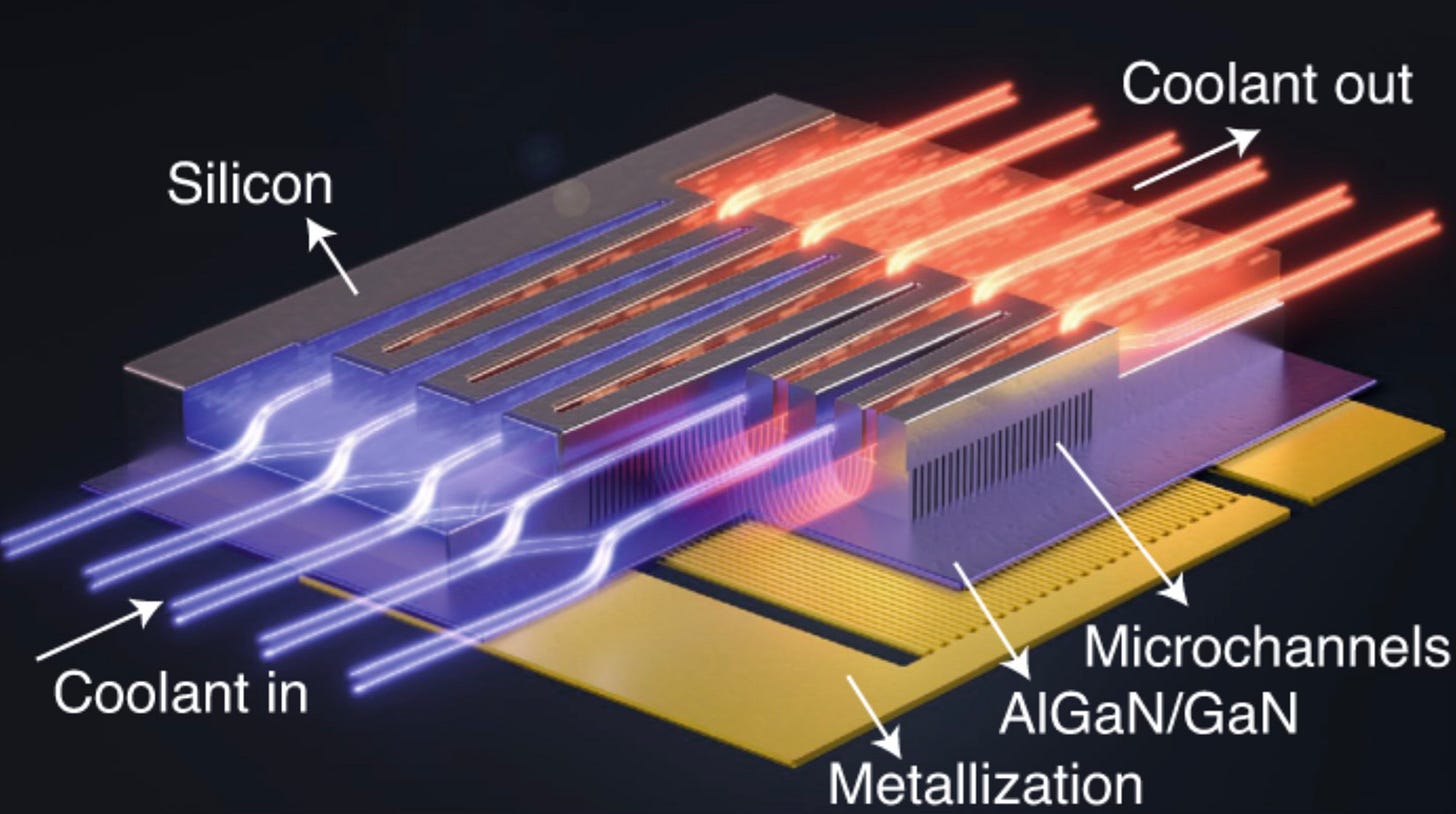
Upstream we’re seeing wild experimentation. In AI-based middleware we have LangGraph and Wordware both helping developers create sophisticated AI agents. Apps like Cursor help coders work faster, Granola simplifies meeting notes and Elicit speeds up literature reviews for researchers.
AI promises to lower the cost of intelligence — a core input in modern economies. When agents can do a task, they do so at one-thirtieth of the cost of a human.
This combination of adaptability, rapid advancement and cost reduction positions AI to join the ranks of historic general-purpose technologies. But AI is being touted as much more than that – and the expectations of the market are resting on it. So, what would have to happen for it to disappoint?
Framing the criteria for “disappointment”
For AI to succeed as a GPT, it must drive a noticeable increase in productivity growth. History shows that this can take time and follows an S-curved trajectory. In the short term, the impacts of a GPT are marginal across an economy, although individual firms can do well. In the medium term, as the technology becomes more widespread, firms are able to retool around the technology and a plethora of upstream and downstream businesses emerge. This all drives growth. In the long term, a technology’s contribution to productivity growth flatlines as it becomes normalised.
In other words, it’s the medium term which matters, the point at which a technology is far enough dispersed into firms in an economy to have a systematic impact on productivity. In the case of electricity and the PC, America start to see GDP growth increase starting in the 1920s. The technology had been commercialised for 30 years by that point. But it started having economy wide impacts when it about a quarter of homes were electrified.
Economists are rather split about what AI might mean to us economically. There’s a wild range of estimates looking at labour productivity growth alone.
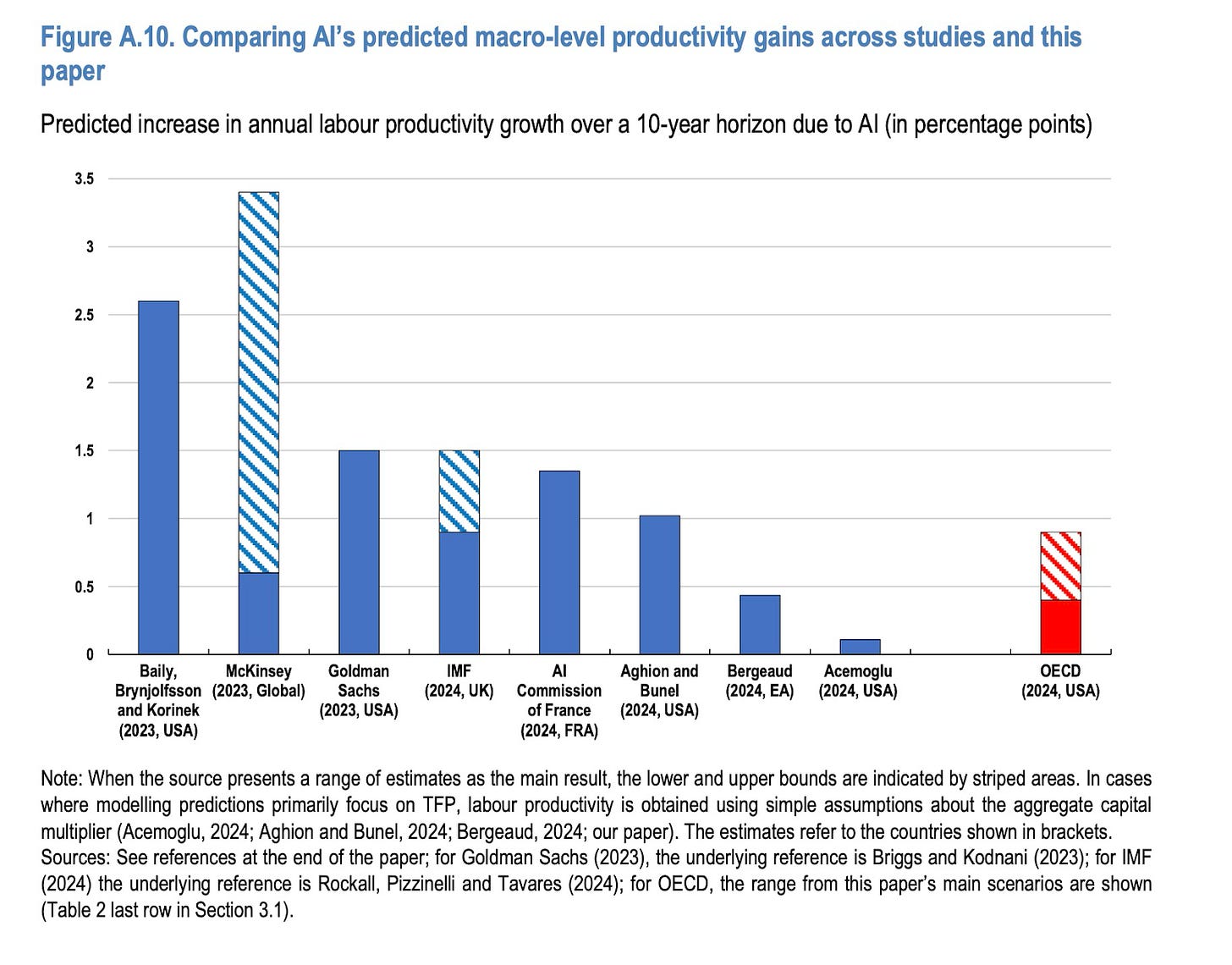
What do those numbers mean in terms of GDP growth? Goldman Sachs, for instance, estimates a 1.5% increase in labour productivity over 10 years for 2023. To make sense of this, let’s turn it into real dollars. US GDP was $27.7 trillion in 2023. This could mean that by the end of the tenth year, US GDP would be between $6 and 7 trillion dollars higher than it otherwise would have been, yielding a cumulative $25-30 trillion dollar increase over the decade.1
For this thought experiment, I’ll define “disappointment” as AI failing to boost labour productivity by 2% by the year five, starting the clock in January 2023. I’m choosing 2% as it’s above most estimates, January 2023 as it’s roughly the ChatGPT starting clock; and five years because many of us, myself included, have argued that AI should get to its point of impact faster than the PC or electricity, which took 15 years and 30 years respectively.
My main line of thinking is that productivity impacts follow the deployment of a technology across the economy and AI can get deployed much faster than the railway: we’re richer and much of the underlying infrastructure, internet and computers, is in place. In addition, the organizational dimensions of the modern economy favor fast adoption. Firms have reorganized themselves dozens of times in the past decades and many of you will have lived through transformation after transformation. This might suggest firms have the capacity and managerial expertise to move quickly if they need to. In addition, capital markets could be more punishing – providing a negative incentive – to slow movers.
Translating that back into numbers, it would mean adding a cumulative $2.5-$2.7 trillion of US GDP between 2023 and 2028, with US GDP about a trillion and change higher in 2028 than it might otherwise have been.
The slow unwind
In the scenario, I am going to outline below, the productivity impact of AI could be limited by several key challenges, even as AI companies themselves grow rapidly. The deployment of AI across the economy might not translate into the broad-based gains we expect, at least not within the five-year timeframe starting January 2023. Let’s unpack why this might happen.
Even as AI companies surge, the technology’s productivity impact could falter due to adoption hurdles. Small firms—startups and agile players—adopt AI swiftly, using tools like ChatGPT or Cursor to enhance efficiency or innovate. Yet, their limited scale curbs broader economic influence; in the U.S., large firms with over 500 employees drive 70% of GDP. If AI’s reach stays confined to smaller entities, it won’t lift aggregate productivity significantly.
Conversely, large firms—the economy’s backbone—often lag in fully embracing AI. Instead of overhauling operations, they opt for modest gains, like automating customer support or refining HR processes. Integrating AI into legacy systems, retraining staff, and restructuring processes prove daunting, slowing transformative change. A retailer might tweak inventory with AI, but reimagining entire operations could take years, yielding only incremental benefits short of AI’s hyped potential.
Compounding this, lackluster results could rattle capital markets. In 2024, investors funneled $320 billion into AI, expecting substantial returns. If productivity gains stall, confidence may wane, mirroring past tech bubbles like the telecom crash. Reduced funding could then hinder AI development, creating a self-reinforcing slump: less investment, slower progress, and fading enthusiasm.
These dynamics—uneven adoption, large-firm caution, and market jitters—might prevent AI from boosting labor productivity by 2% by 2028. This could leave U.S. GDP $2.5–$2.7 trillion below projections for a true general-purpose technology. Though AI may still spark innovation, its economic revolution could unfold more gradually, disappointing those awaiting a seismic shift akin to steam or computing.
Let’s dig in.
Minnows move, mammoths don’t
AI products like ChatGPT and Cursor have seen impressive uptake—ChatGPT reaches 400 million weekly users and Cursor is generating $100 million in revenue within two years.
These successes – and they are huge – are successes for the entrepreneurs building these business. But it is still small beer against the mighty $29 trillion US economy. Thirty years into the internet boom, the digital economy contributes 10% to US GDP. Expectations for AI are much, much higher.
Anthropic and OpenAI forecast collectively $65 billion in revenue by 2027. Assume they grow 30% to 2028. This would take revenues to $80 billion, of which perhaps two-thirds, so roughly $50 billion and change. Could the rest of the sector add several hundred billion dollars of revenue in that short period of time? David Cahn at Sequoia Capital reckoned that Ai firms would need to find $600 billion on revenues to justify 2024’s investments in data centers.
More intelligence than they can handle
Even if AI becomes inexpensive, the economy may struggle to absorb vast amounts of “intelligence.” We’re already seeing this happen. Large companies, who make up the bulk of the real economy, are starting their genAI implementations to eke out efficiencies from low-value uses, like customer support.
As competition in the AI industry spurs more innovation, more and better machine intelligence will be available increasingly cheaply. Look at the price drops we’ve seen in GPT-4 tokens.
Or that OpenAI’s Deep Research product was available at $200/month but is now only $20/month – in just six months!
But companies and industries need to absorb this intelligence. And the economy might have natural saturation points that limit uptake. It took companies, and then industry, two decades to change their processes to make use of electricity in the factories. Even the humble typewriter took roughly two decades to be absorbed into companies.
If AI is as powerful as it might be, integration into firms could be more complex, not simpler. This complexity arises because truly transformative technologies often require sophisticated supporting infrastructure and organizational capabilities to unlock their full potential.
By analogy, consider the Trent XWB engine developed by Rolls-Royce for the Airbus A350. This engine is an engineering marvel, a high-bypass turbofan that delivers remarkable fuel efficiency while generating over 97,000 pounds of thrust. Its advanced materials, precision manufacturing and complex digital management systems represent the culmination of decades of aerospace innovation.
However, to make use of this technology, you need much more than just the engine itself. You need a sophisticated airframe specifically designed to accommodate its mounting points, weight and thrust characteristics. You need specialized maintenance systems, trained technicians and a global supply chain for replacement parts. You need airports with appropriate infrastructure and a ready supply of the right type of fuel and lubricant. Dropping one (in a time machine) to pre-reformation Europe would yield very little benefit: they simply wouldn’t know what to do with it.
This isn’t to say the individuals in the large firms, which make up the bulk of the US economy, wouldn’t start to make smart use of AI. There is already evidence they are. Indeed, a recent survey of surveys by the Fed suggested that worker-level use of AI was higher than firm-level use.
But real change requires the organization itself, and its internal structures from work groups to departments and inter-departmental processes to adapt to the technology. It might need years—or more—to change their internal patterns.
And much of that is true because we’re not just dealing with standard intelligence. LLM intelligence is fundamentally different. It has a ‘jagged frontier’ where it excels brilliantly at some cognitive tasks yet fails unpredictably at others. In situations where AI tools are being used as augmentations to workers, training them to deal with the jagged frontier might delay roll-outs. For developing highly automated systems, higher reliability requirements might restrict activities to well within that frontier.
And even if we did have unlimited intelligence without these issues… How many firms would really benefit today from a thousand PhD chemists showing up at their door? Anthropic’s Dario Amodei might be able to deliver a ‘country of geniuses in a data center’ in a few years, but which companies could actually make use of them in the short term? Only industries that are already heavy in R&D, like pharmaceuticals, might salivate at the prospect but most industries don’t really know what to do with the capabilities.
Systemic constraints—regulatory approvals, funding cycles or workforce skills—further limit how fast cheap intelligence boosts productivity. An AI system designing breakthrough drugs, for example, still relies on clinical trials, approvals and manufacturing.
The bubble bursts, investors flee
The AI boom is driven by massive capital expenditures. Amazon, Microsoft, Google and Meta are projected to drop $320 billion on capex this year, with AI chip demand expected to grow from $114 billion in 2024 to $280 billion by 2028, according to Morgan Stanley.
Morgan Stanley roughly estimates $520 billion by 2028. Assuming a 50% margin, it implies $1 trillion in new revenues that need to be found in that year, well above my threshold for disappointment.
What happens if revenues don’t show up to support those investments? For now, Wall Street is happy with the big tech firms using their balance sheets to fund AI capacity expansion. They’re banking on the promise.
Alongside Big Tech’s balance sheets, hundreds of billions of dollars of financing will come from debt markets to buy the land, build the buildings and finance the power generation these data centers need.
A perceived slowdown in revenue growth could be enough to shatter investor confidence, leading to a stock market collapse and liquidity problems for debt-financed projects.
When the debt-fuelled telecoms bubble burst in the early 2000s, failing firms left behind a vast network of “dark fiber” cables, ducting routes and physical infrastructure that became a critical resource for subsequent internet growth. The OECD noted that while painful, this infrastructure legacy became the “necessary substrate” that enabled streaming, cloud computing and modern AI systems that now consume 85%+ of the original bubble-era capacity.
Unlike the telecom bubble, where unused fiber ducts retained value, high-end GPUs can depreciate quickly – between 2-5 years depending on usage. Those GPUs and data centers may not be useful if there is a bust.
So perhaps a better parallel is the fracking bubble which investor Jim Chanos replays on
’s SubstackSo far, AI is doing the opposite [of the internet boom]. It is a massively capital-intensive business. Someone joked that the top tech companies are now looking like the oil frackers did in 2014, 2015, where more and more capital is chasing arguably a variable return. […] The numbers are now getting so large from just even a couple years ago that the returns on invested capital are really now beginning to turn down pretty hard for these companies.
If the capex bubble bursts—with Big Tech spending approaching $200 billion in 2024 alone—it is unlikely to be contained in the balance sheets of the big firms. With 65% to 80% of data center development funding reliant on debt, a wave of defaults or write-downs in this debt could deepen the crisis, as jittery investors pull back from riskier assets. It will reduce investor confidence in the growth rates of the tech sector, dragging stock prices – and, via wealth effects – consumption down. A real economic slowdown would mean that job cuts would further impact the economy.
Historically, markets take several years, not less than five, to recover from a real asset bubble, suggesting that the tech sector and broader economy could face a prolonged period of instability and adjustment.
Assessing the scenario
As I was writing this essay and testing scenarios quantitatively, I really started to persuade myself that this is far from total fantasy. That a great deal is levered on what we might consider “venture class risk”; the bet that young startups can grow very quickly… Which means they sell their products to the real economy.
But venture class risk has turned into broad stock market risk, because 30+% of the US stock market now rides on tech firms astride the genAI wave. And it ekes into debt markets because genAI is triggering so much infrastructure build-out. For want of a nail, we might say.
In this world, AI’s productivity impact could lag due to slow adoption, limited demand elasticity and over-investment risks. While AI might still yield remarkable innovations and companies, these may not coalesce into economy-wide gains within a decade.
There are other dynamics I didn’t get into. For example, what if the US can’t get enough power infrastructure on stream to meet enterprise demand for genAI? I touched on this risk in an op-ed in the New York Times last year. That would likely result in price rises or supply constraints that could slow down getting results from AI. Or what if genAI adoption in the main economy goes faster than expected and results in significant job losses and a decline in consumer confidence. The negative impact on spending (and increase in welfare costs) could cast a pallor over the economy.
Today’s essay outlines a plausible, low-probability scenario for AI having slower than expected effects on the economy resulting in a cascading disappointment.
It is my baseline view that AI, on its current iterative trajectory, will move faster into the economy than previous technology waves.2
Faster, by the way, does not mean instantaneous. Think in the order of five years rather than a decade plus.
I’ll continue to look for evidence, think through this question and update my beliefs.
I acknowledge I have made tons of simplifications here. These have been introduced to make the essay readable!
A recent analysis by the St Louis Fed provides some evidence, reckoning that workers using generative AI were already saving about 5.4% of their team each week. When averaged across the whole workforce, this was translating to 1.4% of time savings.
I think this is interesting in terms of your last piece also. If the EU is going to go through a continent-wide military renaissance, is it possible AI can be used to organise, standardise and devise equipment and structures in terms of optimum military readiness to maximise freedom and protection through applied intelligence? It's quite possible that all markets could atrophy in a strong military scenario, and prospects for the technology be delayed for commercial purposes, but accelerated for war. The irony would be so heavy if the computer, devised as a tool for war by Alan Turing, could become the architect of European (or other) security nearly a century later..
I'd tag this one. It could be your best red-eye cant sleep need to waste wheels up to wheels down work product!.
From one perspective your analysis was totally needed, maybe over due.
It pretty much captures each of the broad rear-view facing entropic tech evals of the past few biggies (PC started in 84, (Apple was 78), '94 network/Internet, 08 Iphone - data/voice/phones. I do not believe any of these would be a relevant comparison to AI's breadth or depth of reach in TODAY's soci-eco-tech environment. Each of these are "layers"...
What happened?
Each tech shift is of course preceded by the 'EOH' warnings; End Of Humanity. Each of those (above) has given the same EOH NOISE, but signal is available for fine tuned listening. AI is out of the barn! It's about getting in the game. When there was no bandwidth, construction exploded, no data centers, investment exploded...no cell towers, can I lease your Roof... :)
Along the way there was unexpected, unanticipated, exponential growth in new concepts, new business categories, new markets, scads of new revenue showered into the economy.
The predictions were chaos, entropy consumes the fragile human species, dust.
NO ONE...ever thinks any of those EOH diatribes...
WHY?
Because only a loonatic can imagine anything as wild and creative as what is about to come. Robots? flying cars? no answering services? no IRS dipstick? No 3hour hold times...somethings are heaven on earth!! But, someone would have to be nuts to pontificate very loudly, because the amount of change that is coming is big!
Can you imagine...some nut SAID HE'S going to CATCH a 400foot tall, 40000ton ROCKET!! hahaha...hes' a nut...right! :)
These type of people, the creators, the lunatics, when they speak, and act, it does one of two things...
CREATIVITY: The future engages your creative energy; anything is possible thinkers!!
OR
FEAR: It ignites base human FEAR. For those who are very unsettled by "Change" (a word synonymous with "life") thoughts about life altering, inevitable, no way out, change? For those who do not cope well with change/life, yes, these are significant enough to engage their Base human fear - being worthless, being invisible, or more invisible, being replaced, etc.; Ultimately, fear of being unlovable. These are the "oh shit we're gonna die" folks!
If there was no concept of "change", we arrived at today, 2025 and that's it!! In a coupla' years, 2028.... "its" over...
Yes, every point you made in the near term is valid; 100%.
The good news is there is a abundant supply of creatives and the entropy perspective never yeilds fruit. This makes sense. Creativity is required for human survival.
The logic would render to: Create or Die.
Your analysis is species wide so I don't see it shaking out the latter Az.
It was a good piece.