In Part 1, we talked about how the volume of scientific knowledge limits individual researchers and traps knowledge in silos — and how AI is emerging as a valuable partner…
If you haven’t read Part 1, I recommend you start there.
Creation: A catalyst for new knowledge
Knowledge, we argued from Part 1, is growing exponentially, at 5% a year. Data is also growing exponentially—with the world set to reach 175 zettabytes of data by 2025. This volume would take 1.8 billion years to download using the average internet connection!
How do we keep up with all this information and turn it into something valuable? Our individual cognition is limited: research suggests that we humans can only hold four variables in our working memory.
Fortunately, we have various tools to amplify data-analysis capabilities, including calculators, spreadsheets and statistical software. Among these, AI has emerged as a significant and transformative addition to the data analysis toolkit. AI stands out as a two-pronged tool: on one hand, acting as a robust microscope, delving deep into vast data sets to unearth hidden patterns, and on another, it’s an accelerator, speeding up our transformation of data into knowledge.
A microscope on hidden interactions
The invention of computers has helped us process large amounts of data faster and more accurately. But we’re left with one problem—the operators (i.e., us) still have to make an ‘educated guess’ (or hypothesis) about how something works. Then, we’d examine the data to confirm or reject this hypothesis. But with all the complexity in the world, it’s unrealistic to think we can fully grasp all the interactions and insights within the data.
Machine learning helps to solve this problem by enabling data-driven discovery. Computers can pinpoint patterns in large data sets that elude human observation and therefore guide hypothesis formation.
Deep learning, a specialised subset of machine learning, elevates this process. It employs layered neural networks, mimicking our brain’s information processing, to progressively extract intricate data features.
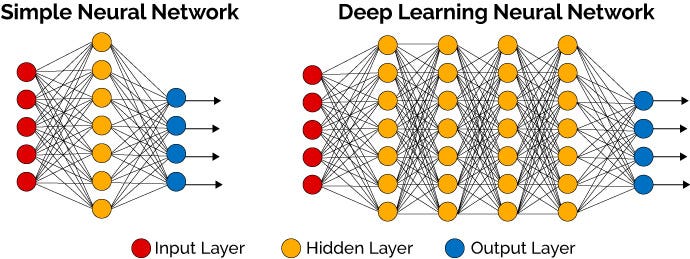
Take earthquake detection, for example: early neural network layers might recognise basic waveforms, while deeper layers can differentiate earthquake types and origins from background noise. The diversity of applications is vast: deep learning has been used to predict protein structures (see AlphaFold), map crystal-structure phases and even discover new classes of antibiotics.
While tools like AlphaFold are groundbreaking for figuring out protein structures, we must be cautious about over-reliance on machine learning. These systems excel at some tasks, but there are other important areas of science which are harder to model with AI, and we mustn’t neglect these nuances. For example, proteins aren’t just still objects; they move and interact. They are dynamic ones undergoing conformational changes from environmental factors; they interact with proteins and ligands to form new complexes; they may attach to specific nucleic acid sequences; and they might undergo post-translational modifications, like phosphorylation. This peripatetic lifecycle is not something AlphaFold fully captures. Overusing machine learning could mean we miss out on the bigger picture.
Recent advancements, like Bioptimus’s general biological generative models, mark a shift from single-faceted approaches (e.g., AlphaFold’s protein structures) to analysing multimodal biological data. This enables the capture of intricate biological interactions by incorporating diverse data types.
Furthermore, projects like Evo, a DNA foundation model, break new ground by analysing long DNA sequences while remaining sensitive to minute changes (e.g., individual nucleotides). This means Evo can not only grasp interactions between various biological components but also comprehend how these interactions unfold across the entire genome, leading to a richer and more nuanced understanding of biological systems.