How quickly will it be before artificial intelligence systems can undertake very long tasks without human intervention?
This is a question with massive implications for productivity, automation and the future of work. A recent analysis from METR Evaluations suggests that AI’s ability to sustain task execution is improving at a fast rate. The length of tasks AI can autonomously complete is doubling every seven months. If this trend holds, by 2027, off-the-shelf AI could handle eight-hour workdays with a 50% success rate.
Imagine if a factory worker could only work in 10-minute shifts today but, within three years, could complete a full 8-hour shift without breaks. That’s the trajectory AI might be on.
That’s a bold claim. And it’s not just coming from outside observers—insiders at major AI labs, including Anthropic co-founder Jared Kaplan, have signalled similar expectations. I take their claims very seriously. They have access to the models, the data, and the emerging scaling trends. And in both formal discussions and casual conversations, 2027 (or sometimes 2028) keeps coming up.
What does this mean? If AI can reliably execute long tasks, it reshapes industries, from knowledge work to automation-heavy fields. But is the trend as inevitable as it seems? And just how reliable do these systems need to be before they become truly transformative?
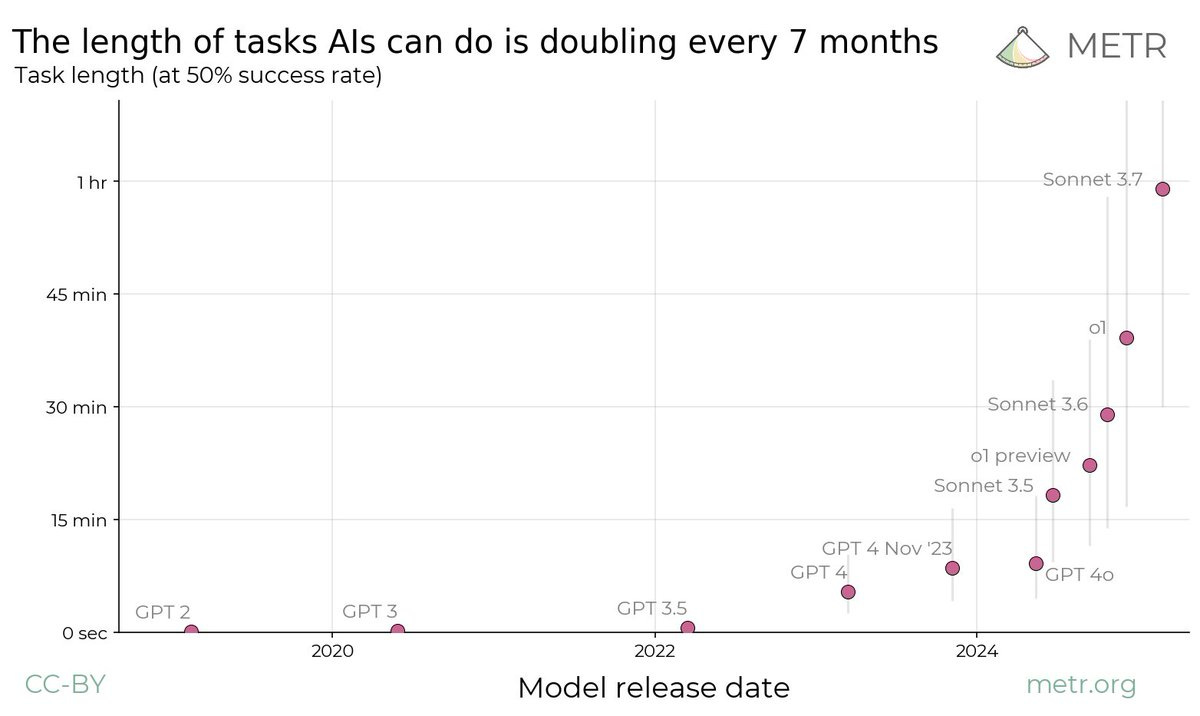
The idea of task length and success rate is an important one. GPT-3 was good at two- or three-second tasks: it was pretty reliable at pulling out the nouns or entities in a sentence. GPT-3.5 could get to a few tens of seconds; in a mediocre fashion parse a paragraph. Those of us using o1 or Sonnet know that we can throw much more complex tasks at them.
METR put clear bounds on the nature of this test:
The instructions for each task are designed to be unambiguous, with minimal additional context required to understand the task… most tasks performed by human machine learning or software engineers tend to require referencing prior context that may not easy to compactly describe, and does not consist of unambiguously specified, modular tasks
What is more:
Relatedly, every task comes with a simple-to-describe, algorithmic scoring function. In many real world-settings, defining such a well-specified scoring function would be difficult if not impossible. All of our tasks involve interfacing with software tools or the internet via a containerized environment. They are also designed to be performed autonomously, and do not require interfacing with humans or other agents.
METR’s research focuses on modular, well-defined tasks with clear scoring functions—conditions that don’t always apply in real-world scenarios.
How generalisable do the tasks need to be? AI systems already excel at tasks—such as chess analysis or anomaly detection—that would require hours of human effort, completing them in minutes. Just think about Stockmaster, the chess engine. Or any of the ML systems that detect anomalous patterns in financial data. METR has chosen rather more generalisable tasks than those narrow cases.
What level of performance do we need? A 50% success rate isn’t really a like-for-like comparison with human effort. While humans are far from perfect, in most work situations we are probably aiming for greater than a half chance.
One Twitter X user visualized METR’s data, plotting accuracy rates of 80%, 95%, and 99% on a log scale. The results show a clear trend: lower accuracy thresholds improve rapidly, while reaching near-perfect performance (99%) follows a much slower, more effort-intensive curve. This reinforces the challenge of achieving high reliability in AI outputs.
It’s a Pareto relationship. Reaching 80% accuracy is relatively rapid, potentially viable for four-hour tasks by 2028, while achieving 99% accuracy demands exponentially more effort, pushing timelines further out. This disparity shapes expectations for AI’s practical deployment.
Even a system that is fast, cheap and 50% accurate can be a game changer—provided we can quickly check its work. If it has made a mistake, we can then ask it to do it again or send it to a system (like a human) which might be slower but more reliable. Of course, if we can’t evaluate its work cheaply, then the cost of evaluations (in dollars or time) may make it uneconomic.
On the other hand, something that is 80% accurate would seem like a fair building block of a solid system.
The inexorable economics
I did some napkin math for a notional four-hour task.
Assuming each task requires 1,000,000 tokens at roughly $10. (Token costs are coming down, but let’s just use that for now.)
Each task has to be verified by a human, perhaps using some formal verification software. That verification takes 15 minutes.
If the task is not done correctly, the human needs to do it. This takes four hours.
The human’s fully loaded cost is $100 per hour.
To do 1,000 of these tasks manually costs 4,000 man-hours or $400,000.
What about the AI?