🔮 The Sunday edition #499: Biology’s bottlenecks; the AI economics & productivity; bitcoin high; human rhythms, voting & organ ageing ++
An insider’s guide to AI and exponential technologies
Hi, it’s Azeem. Welcome to the Sunday edition of Exponential View and thank you for reading! Every week, I help you ask the right questions and think more clearly about technology and the near future.
💬 I am sorting out my speaking engagements for Q1’25 – now is the time to get in touch if you want to bring my perspective to the table. I have a couple of slots left, so reach out.
Ideas of the week
Facing the turbulence
The exponential transition to an abundance of energy and intelligence will not stop regardless of who is in the Oval Office. Yesterday I wrote about the dynamics that can help us overcome the turbulence in the coming years.
The most interesting areas of industrial innovation and strategic autonomy are in climate tech — zero-carbon materials, the new energy system, the bioeconomy and new industrial techniques. Climate change adaptation and mitigation require coordinated action. In an administration that might lack an interest in coordinating, technology may be what creates the space for policy and action.
The biology paradox
We need better AI to accelerate biological research, but we first need better biological tools and data in order to build effective AI models. As bioengineer and writer
points out in his exceptional commentary on Anthropic CEO Dario Amodei’s prediction that AI could compress a century of biological progress into a decade, the reality of AI and biology is complex. Even our most celebrated AI breakthrough, AlphaFold, reveals the gap between static prediction and biological reality: while it can predict rigid protein structures, it fails to capture the constant shape-shifting of proteins during biological processes, their intricate interactions with other molecules or their context-dependent behaviours. Whether AI and simulation can capture this complexity is yet to be seen. The scale of our ignorance is humbling – even E. coli, our most studied microbe, has hundreds of genes with unknown functions. Our current tools capture only static snapshots of a wildly dynamic system that spans ten orders of magnitude, from atomic bonds to whole organisms. If we can’t simulate the whole dynamic process in a computer, we have to experiment with it in reality. This is where we’re bumping up against Amdahl’s Law: a process is only as fast as its slowest required step. The tedious pace of experiments (cells simply take time to grow) becomes the limiting factor. To break through, we need to solve fundamental wet lab bottlenecks, not just improve code.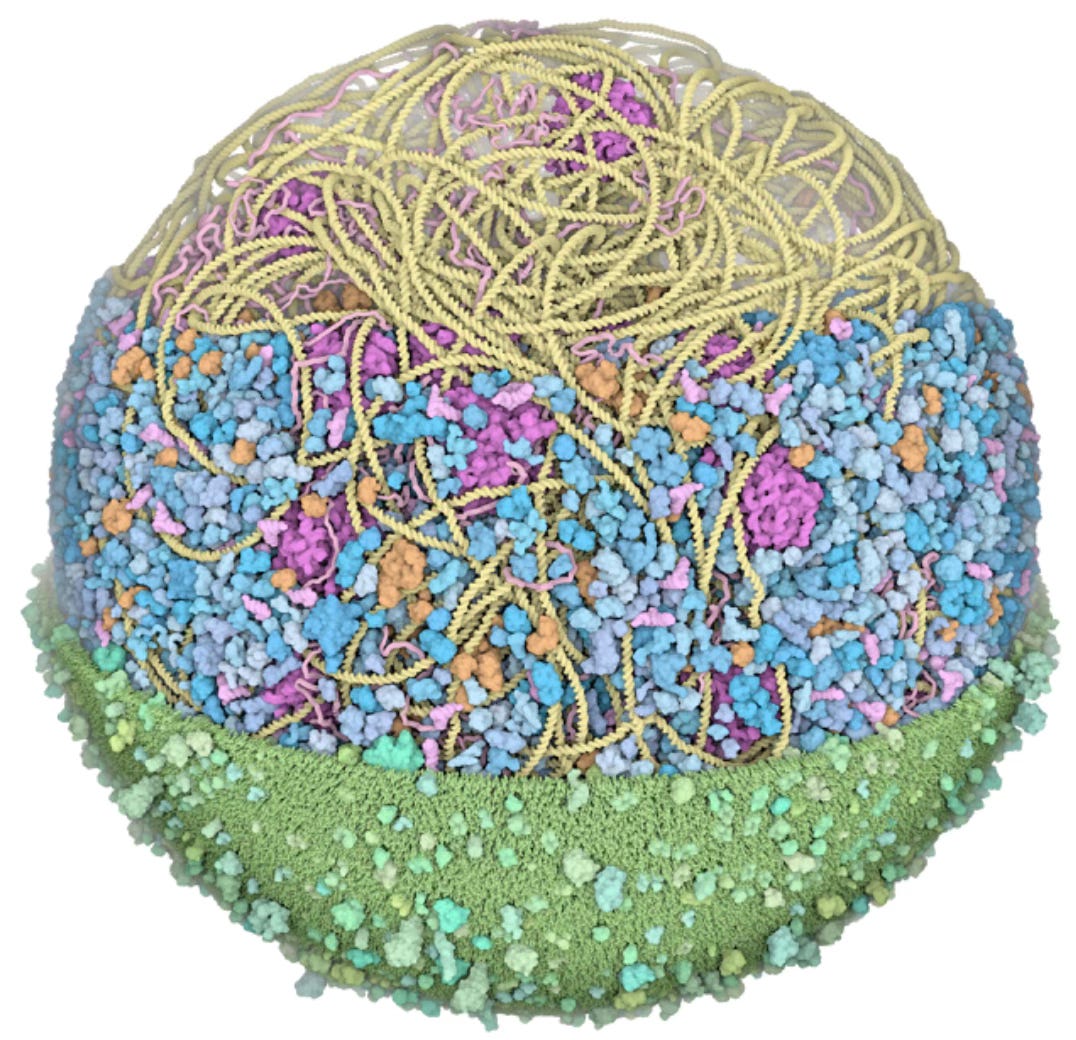
The economics of AI
A recent observation by Marc Andreessen that more compute isn’t yielding proportional intelligence gains, aligns with what we’ve long known about scaling laws: we need exponentially more resources for linear gains in performance. While we haven’t hit the technical ceiling yet – and OpenAI’s upcoming Orion model might demonstrate meaningful gains – the real constraint may be profitability. Training and running these massive models costs hundreds of millions, even billions of dollars, making it a game that only the biggest tech companies can play (see the State of AI report for just how large these clusters are getting). During my recent trip to Silicon Valley, I found a strong belief that scaling remains. But, here’s the catch…