Somewhere, something incredible is waiting to be known. — Carl Sagan
Science helps us understand the world around us. It uncovers the secrets of the universe, from atoms to stars. Science enables the creation of knowledge and discovery that changes how we live and see ourselves. And there is a lot to be known! Thousands of diseases are still without a cure, 10 million people die of cancer every year; we have just scratched the surface of how our own brains work; and the human-made crisis of climate change needs more knowledge, not less, to prevent the worst outcomes and rehabilitate damaged ecosystems.
However, our minds have limits. Individual humans can only see, understand, and remember so much. Could AI techniques help accelerate scientific discovery by addressing the limitations of human cognition?
Answering this question is the focus of this Chartpack. It will come out in two parts:
In Part 1, you will learn how AI improves the way we access and connect existing knowledge.
In Part 2, you will learn how AI-powered tools are transforming real-world scientific research and development, fuelling a new era of innovation.
Communication: Unlocking existing knowledge
Knowledge-sharing provides access to the wisdom and experience of others without the need to reinvent the wheel. However, there is a fundamental problem: humans have a limited capacity to store knowledge, while the amount of knowledge is growing exponentially, estimated to double every 15 years.
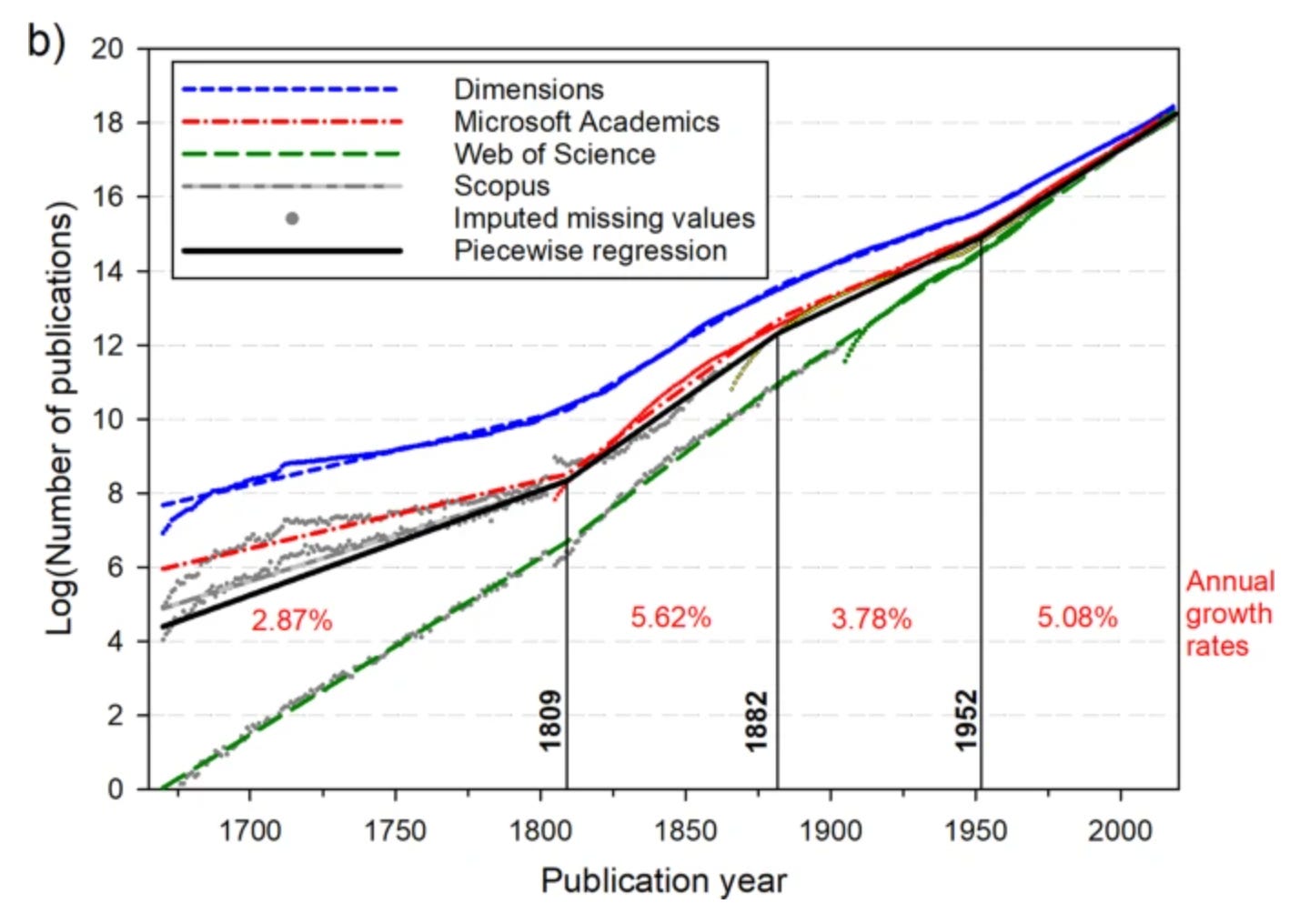
This increase in knowledge, combined with professional, organisational and financial incentives in academia, has led to extreme specialisation, and increasingly niche research — akin to putting blinders on a horse. According to one estimate, 45% of research in the humanities is never cited. This leads to hidden public knowledge, where valuable insights remain trapped in academic silos, inaccessible to the wider public and even to fellow researchers.
So there is a dual problem to be solved: (1) How can we improve access to knowledge, and (2) How do we improve connections between existing knowledge?
Finding knowledge
Tools have always shaped the sharing of knowledge. The printing press and the subsequent development of mass media allowed knowledge to be distributed and stored on a massive scale, while libraries helped us organise it. Then came the Internet and search, allowing almost instant access to a seemingly infinite number of potential sources of information and data. However, Google and similar search engines often leave it to the user to convert this raw information and data into usable knowledge.Â
LLMs can solve this problem. In fact, back in 2020 — four years ago —
wrote about then-SOTA GPT-3…What GPT-3 has achieved is to encapsulate knowledge, billions of words of it, in a sufficiently parameterised model that it can give granular answers to very different types of queries, across multiple domains. It can also follow quite complex instructions across those domains.
Tools like Perplexity, System and Elicit offer the next leap in the way we gather and synthesise knowledge. As Aravind Srinivas, co-founder of Perplexity, said in his chat with Azeem:
The moment you submit a query, we’re doing the work a typical person browsing the website would have done. Imagine the equivalent of human labour in trying to write a Wikipedia article for the question you asked but done in one or two seconds and on-demand 24/7. Dig deeper, ask clarifying questions, and ask any number of questions without judgment.
Perplexity condenses information from multiple sources into clear summaries, reducing the time it takes to find relevant knowledge. System & Elicit, on the other hand, helps speed up the literature review process by allowing researchers to find relevant papers more easily, read AI-generated summaries of abstracts tailored to their needs, and systematically extract key data points. Other tools can even speed up meta-analyses, such as Pitts and Laser AI. These tools make finding Sagan’s incredible just a little bit faster.
Connecting knowledge
Even with increased access to knowledge, the challenge remains: how can we effectively make connections between different fields and gain new insights? One powerful approach lies in using AI to ‘T-shape’ individuals: to develop deep expertise in one field alongside a breadth of knowledge across others. T-shaped professionals can rapidly grasp fundamentals across various fields and foster the kind of interdisciplinary thinking that drives innovation. In Geoffrey Hinton’s words:
[AI] is very good at seeing analogies. What’s more, it knows thousands of times more than we do. So it’s going to be able to see analogies between things in different fields that no one person had ever known before. That may be this sort of 20 different phenomena in 20 different fields that all have something in common. GPT-4 will be able to see that and we won’t.
AI will be able to see the hidden connections within existing knowledge. Historically, many innovations have demonstrated this potential, such as the fusion of insights from biology, observing the adhesion mechanism of burdock burrs, with materials engineering and textile technology to create the brilliant invention of Velcro (bye-bye shoelaces).Â
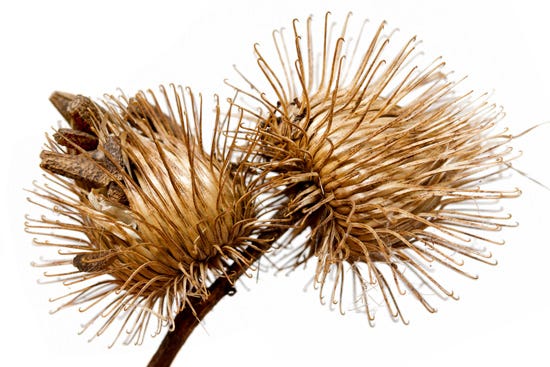
In the AI domain, Markus Buehler’s research on MechGPT is an illustration of how LLMs could be used to uncover hidden connections within existing knowledge. MechGPT is a specialised LLM fine-tuned on knowledge in multi-scale materials failure. Below is an example from the MechGPT research paper demonstrating how it creates connections across seemingly disparate areas — creative English writing and materials design.
Write a creative story for an English class.
Once upon a time, in a land far, far away, there lived a curious and adventurous young girl named Lily. Lily had a passion for exploring the world around her and was always on the lookout for new discoveries.
One sunny day, as Lily was wandering through a lush, green forest, she stumbled upon a mysterious, glimmering object hidden among the trees. Captivated by its beauty, Lily reached out to touch it, only to find
that it was made of a shimmering, transparent material she had never seen before.
...
News of the mysterious object quickly spread throughout the village, and soon everyone wanted to know more about it. People began to call it "The Dreamcatcher," as they believed it had the power to capture dreams and grant wishes.
...
This is great. Now expand the story to add an analysis of the "Dreamcatcher" via multiscale modeling on large supercomputers.
...
Intrigued by the possibility of unlocking the secrets of The Dreamcatcher, a team of scientists decided to
study it using multiscale modeling on large supercomputers. They believed that by analyzing the object at
multiple scales - from the atomic level to the macroscopic level - they could gain a deeper understanding of its
properties and potential applications.
In the end, after months of analysis and data processing, the scientists presented their findings to the world. They discovered that The Dreamcatcher was made of a unique material with extraordinary properties, which they dubbed "Luminium." Luminium was incredibly strong and lightweight, with the ability to refract light in
a spectacular fashion. The scientists also found that The Dreamcatcher had the power to absorb and store energy from its surroundings, making it an incredibly efficient source of power. This discovery opened up a world of possibilities for new technologies and applications, from renewable energy systems to advanced materials science.
...
Now, hypothesize the specific atomistic and multiscale structure of Luminium.
Based on our analysis, we hypothesize that Luminium is a unique material composed of a complex, multiscale structure. At the atomic level, Luminium is made up of a combination of lightweight and strong materials, which contribute to its extraordinary properties.
At the macroscopic level, Luminium exhibits a distinct crystalline structure, which is responsible for its ability to refract light so efficiently. This crystalline structure also plays a role in the material's ability to absorb and store energy from its surroundings.
In addition to its atomic and macroscopic components, Luminium also possesses a "mesoscopic" structure, which refers to the arrangement of molecules and nanostructures within the material. This mesoscopic
structure helps to govern the movement of electrons and other particles within the material, contributing to its overall properties and behavior.
...
That's amazing. Now, my last question, what atomic elements are the most prevalent in Luminium?
Atomic elements such as Hydrogen, Carbon, Oxygen, and Silicon are likely to be prevalent in Luminium, as they are common in many strong and lightweight materials. Additionally, other elements such as Aluminum,
Magnesium, and Titanium may also be present in significant amounts, contributing to the material's overall properties.
A major limitation of current LLMs is that they are not trained on many academic articles, especially those hidden behind paywalls or within closed-source systems. This restricts their ability to draw on the full wealth of scientific and scholarly knowledge, potentially hindering the discovery of those groundbreaking connections Hinton describes. To fully realise the promise of LLMs, we need more robust open science initiatives to democratise access to research (for one such example, see HELIOS Open).
In the cases I described earlier, you are prompting the LLM to find hidden knowledge, but there is another way: get the LLM to prompt you. Supermind Ideator from MIT Center for Collective Intelligence, co-created by EV member Gianni Giacomelli, does just that. The system acts as a catalyst for creativity. It combines existing problem-solving techniques — such as brainstorming — with the power of AI to generate entirely new ideas. These AI-generated suggestions can either be used directly or as inspiration to trigger further original thinking.
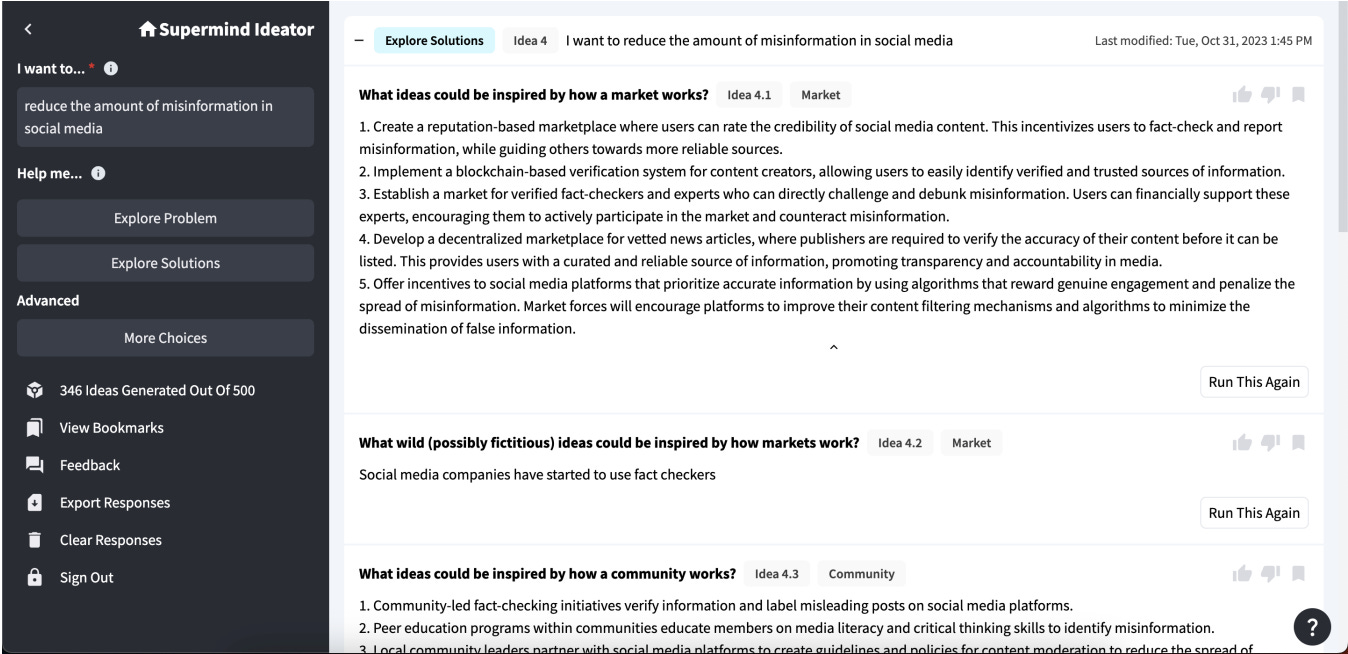
In both cases, LLMs foster connections between existing knowledge, catalysing a synthesis that can ignite human ingenuity and drive the creation of new knowledge. In this way, LLMs have made it a little easier to disseminate and connect existing knowledge. It lays the groundwork for the next logical step: the creation of entirely new knowledge. And AI isn’t just a tool for retrieving and connecting what we already know — it’s a powerful engine for creation. In Part 2 we will be covering how AI acts as a microscope on the vast amounts of data in the world, revealing hidden patterns and accelerating scientific discovery.
And now I would like to hear from you:
Are you a scientist using tools like Elicit or Pitts? Or are you rolling your own? What has your experience been?
Has ChatGPT (or any other LLM) ever helped you make a surprising connection between different areas of knowledge?
Thanks to Gianni Giacomelli, Rafael Kaufmann, Nick Arini & Alan Lesgold for their invaluable feedback on the piece!
this is a great topic - this kind of research about "how" to think and learn is badly needed
I can't recall so far of LLMs helping me to connect entirely different fields; however, the amount of connections that Perplexity has helped me to make within the same field or across adjacent fields is staggering
can imagine that multimodal applications will make unconventional connections even more powerful
super excited about Supermind Ideator and eager to start using it : for strategizing; for product development; for social / political reasoning
Great thoughts:) Not a scientist myself but an engineer building AI-first DevTool for computational biologist. Super excited!